Beyond the Buzz: The Rise of LLMs and AI in Communications Surveillance
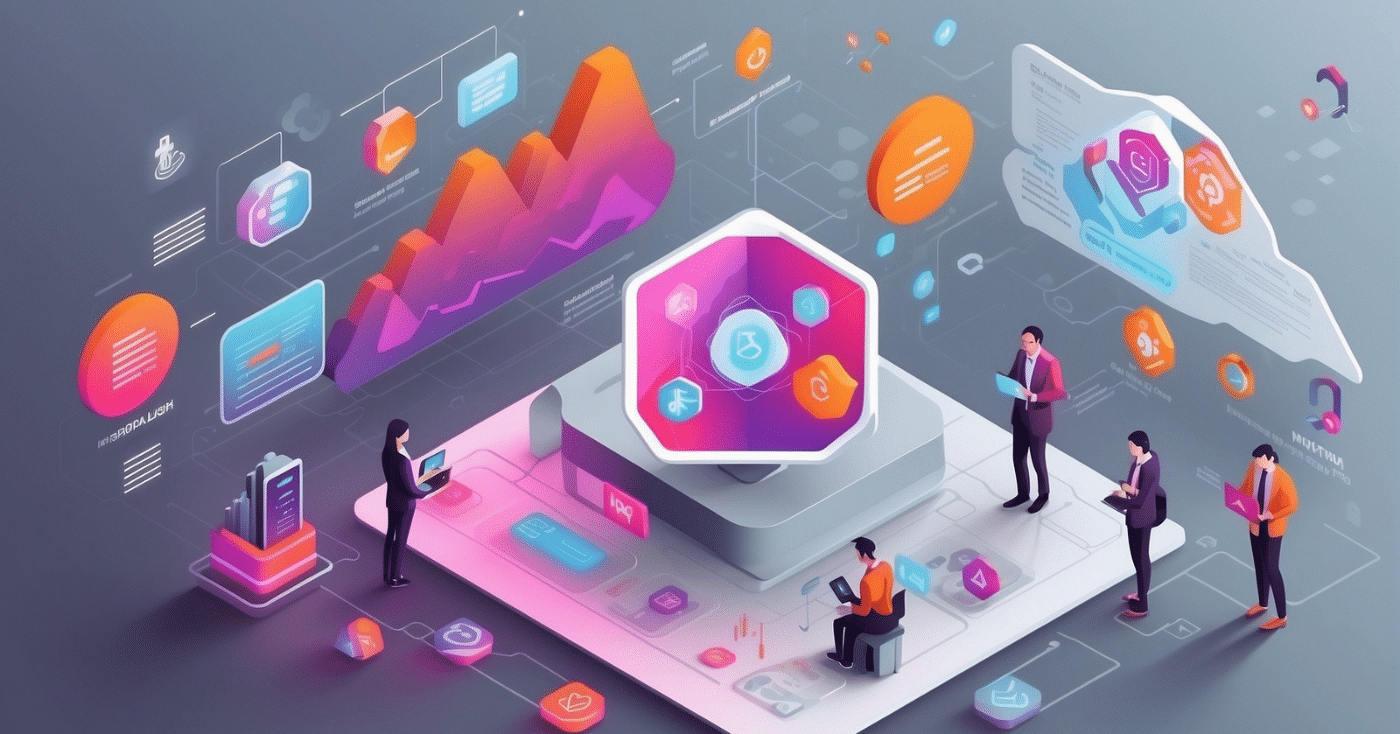
AI has become not only a buzzword but a catch-all for all things tech. But it shouldn’t be. Artificial intelligence (AI) has been around for decades but in the past 20 years it’s become more than just a pipe dream of Stanley Kubrick’s “2001: Space Odyssey,” and more of a reality. One recent example is the rise of ChatGPT: I asked it to describe itself. Its response was, “ChatGPT is a state-of-the-art language model developed by OpenAI. It’s designed to understand and generate human-like text based on the input it receives.”
Basically, ChatGPT is a search engine with more nuance, more brainpower, more human ability to form sentences—and if you’re not convinced yet, well, you should know that everyone else is. In the first two months of launching, the chatbot reached over 100 million users—making it the fastest growing consumer technology in history.
Large-language-models (LLMs) and AI are touching all industries and types of users. It’s not just students desperate for 500 words on “The Scarlet Letter,” executives and traders are also utilizing the tool to make their everyday tasks easier. Now that we know the reality, we must ask, where does that leave compliance?
In a recent webinar, industry experts came together for a lively discussion on the myriad of ways AI is already making many surveillance processes more efficient and how it’s going to continue affecting the industry.
The panel discussion was moderated by David Aaronson, Shield product marketing manager. It featured Dr. Shlomit Labin, Shield’s vice president of data science, who holds both a PhD in psychology and data science and was also recently named “Best Cloud Innovator” in Europe. Key panel guests included Cameron Funkhouser, a retired FINRA executive with deep expertise in regulations and compliance protecting the financial markets and Stan Yakoff, Citadel’s head of supervision for the Americas. The net-net is that there was much to be learned from these experts. So, we’re sharing those insights below.
LLMs – Current State of Technology
Deep learning, powered by AI, is applied to massive data sets towards the goal of becoming enabled to summarize, understand, generate, and ultimately predict which content should be used in the given context. To date, it has been trained on a data set exceeding 3.4 billion words that occupies 45 TB (terabytes) of storage space. Until recently, monitoring human conversations via free language analysis has been one of the biggest challenges facing RegTech vendors and compliance officers. Simply stated – it’s really hard to interpret and infer meaning when people are communicating with each other a couple of words, emojis, abbreviations, and/or acronyms at a time.
Funkhouser offered a bit of context that puts the spotlight on how far the field has advanced. “Back in the 1990s,” he said, “when I was developing ‘innovative’ AI solutions, they didn’t do much beyond analyzing structured text. I find it laughable now to compare how far we’ve come.”
For analysis to be effective, and we’ll use clustered trading as an example, 30-90 days’ worth of activity is the minimum to consider. These longer horizons tend to even out the trading spikes (and noise) that typically occur in response to interest rate bumps, negative daily news, and that sort of thing.
As Yakoff explained, extended analysis periods offer a better sense of “normal distribution” which enables compliance officers to identify anomalies with a greater level of confidence. He said, “Once anomalies are detected, you can shift to the investigation phase to determine what is driving the heightened activity you identified so that you can then categorize it as market abuse – or not. Creating that feedback loop between the front-office and what the surveillance system found enables next-level analysis.”
Trained algorithms can offer interesting insights into the tone of a conversation and its associated emotional context. Of course, much of this is still experimental, just as ChatGPT is. However, we can extrapolate from its operations in generative AI that it is limited as a tool which can inform next steps, but it currently lacks the capability of arriving at a conclusion that can be trusted. Dr. Labin elaborated, “The technology is legitimate but it’s not easy for organizations to adopt it. Some of this resistance is related to the gravitas of the regulations. On the other hand, if your grandmother and toddler can ask ChatGPT for an answer, why can’t you use this approach to intuitively analyze your communication data?”
What the Regulators Want
Here’s the magic answer: They want financial firms to be compliant. Beyond that, regulators aren’t all that interested in what financial firms are experimenting with so long as they achieve baseline compliance.
Funkhouser reiterated that regulators don’t expect perfection. However, they do expect reasonable systems and solutions that you can explain to ensure your approach to compliance is understood. If you can demonstrate predictability and transparency, then regulators will be satisfied. He mused, “Regulators are skeptical, but they also want to see results. If you can show them how you can get ahead of a pending crisis and report it to a regulator before it happens, even if it’s not a perfect solution, they will think you are fantastic.”
Back in the day, compliance officers like Funkhouser literally watched trades happening in the market in real time using a rudimentary computer. Today, that is impossible given unprecedented trade volumes. Yakoff spoke poignantly about the reservations that many firms hold about moving forward. Those who are resistant to technological advances like generative AI often hide behind the cloak of regulators citing that they can’t do A or B because the “Feds won’t approve.”
But generative AI and the sheer size of the LLMs in the market today are making it clear that they are here to stay. And that they offer formidable solutions which cut through all the complexity of querying a dataset until it has been distilled down to something so simple that anyone can get it done. He added, “Where things get a little muddier is how to leverage these sophisticated technologies to address more challenging problems like predicting risk.”
Security and Controls
What the entire panel agreed upon was that the implementation of adequate security measures is currently holding firms back from their ability to be fully autonomous with AI. Shifting from defense to offense is non-trivial. While it is literally almost child’s-play to enter a query into ChatGPT, Bard, or other generative AI models, keeping that data protected and private when it is shared over an open AI is next-level challenging. The second challenge around security is the ability to explain what is happening.
Non-vendors argue that there are fundamental design problems in the RegTech and generative AI tools on the market because the need for transparency is overlooked. The result can be “AI hallucinations” which is a new term but an increasingly common outcome of generative AI, like false citations. Duke University conducted research to demonstrate that ChatGPT invents citations, so it’s not as reliable as many people perceive it to be. At this time, it is also challenging to deduce if the answer provided is original or a regurgitation from something already published.
Dr. Labin raised her concerns, “We need checks and balances, so we know what we have, know what we missed, and know that whatever we produced is correct in addition to needing improved security for the information that we share.” In layman’s terms, each design needs to consider a decision path if something is unclear. Semi-supervised machine learning and confidence intervals are essential aspects of the design. A significant problem is that there are hundreds of regulatory agencies doing so. This necessitates that a system be designed to reflect all those views especially as they change.
On the topic of checks and balances, Dr. Labin and Yakoff discussed the fact that internal controls are critical. “As innovative as each of us are on the path of providing new solutions for RegTech,” Yakoff opined, “there are bad actors out there who are possibly even more innovative but applying their efforts to nefarious practices like scheming how to go undetected by the surveillance systems we are developing. Their goal is to defeat whatever technology you have in place.”
Ethics and the Application of AI
There are plenty of opportunities for companies to upgrade via AI. For example, corporate registrations are still filed on paper in many places. Another opportunity applies to larger companies who typically have more than one job title – and salary – for the same job description. Tightening that up helps the company identify potential issues with unfair labor practices.
The “sister” best practice to data hygiene is data provenance; essentially knowing where the data came from, who touched it, how they changed it, and so on. There are countless dependencies that firms may not even realize they have. Take, for example, all the sub-vendor relationships like Bloomberg, WhatsApp, and other datasets or data aggregation services, as well as the generative AI tools we have been discussing. Where did they get their data from and who really owns it?
On the topic of ethics, keeping up with the volume of eCommunications data exchanged is already incredibly difficult. Exacerbating that problem is the slippery slope of ethics as people no longer deem some behaviors as unethical because of their “the company owes me” mentality. Dr. Labin offered, “Phrases like, ‘don’t text me here’ are dead-ringer giveaways for a compliance officer to investigate further.”
This is precisely where LLMs will have their heyday. If we can use those massive datasets to identify and interpret the nuanced language of someone attempting cheat the surveillance system, we collectively move one step ahead of the bad actors.
AI is Reshaping Reality
Making life easier for compliance officers is goal number one. They are currently inundated with ever-changing and global regulations, ridiculous volumes of content spread across multiple eComms channels, and the increasing use of voice notes as well as the ambiguity of emojis. Dr. Labin stressed that, “LLMs need to evolve beyond words given how the rising generation of youth communicate with symbols, videos, and pictures.”
We are many years away from AI tools being able to make the decisions for us, but we are certainly at a point of technological advancement where we can use such tools to gather information and present it to us in a way that we, as mortal humans, can digest. Officers cannot be co-dependent and surrender all responsibility and accountability to the technology they utilize. They also need to test their systems in place to see if they do operate as defined in the explanatory materials, models, model bias evaluations, and governance documents in place.
It’s no secret that AI is reshaping the world, including our world of eComms surveillance. Based on an audience poll during the webinar, almost 60% responded that their firm was still in the experimental stage with regards to the implementation of AI in their compliance strategy. There is a lot of opportunity still in the industry, and with the right multi-layered approach, teams can expect that LLMs and AI will only continue to supplement their effectiveness.
Related Articles
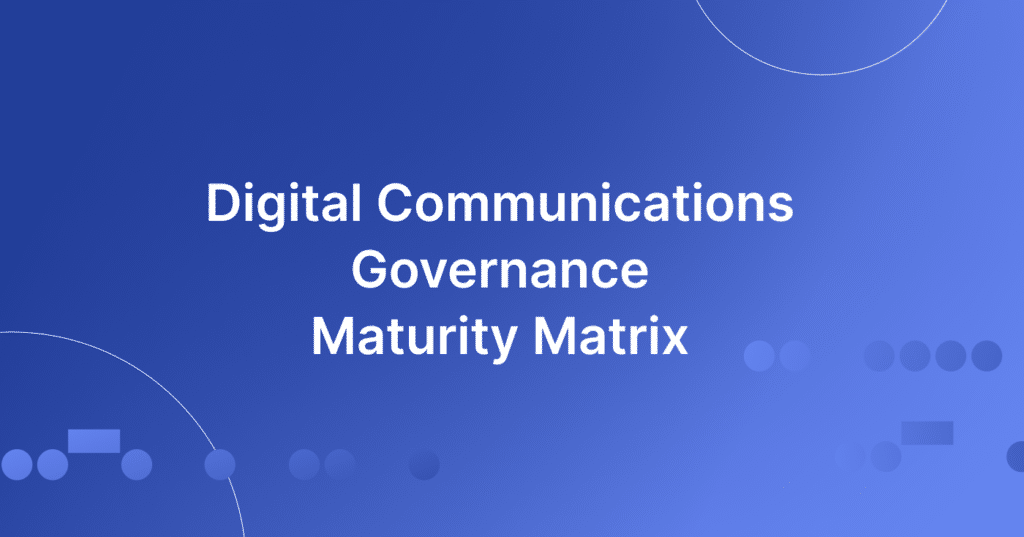
DCG Maturity Guide
Subscribe to Shield’s Newsletter
Capture everything. Deploy anywhere. Store in one place.